Ultrayolo¶
Tensorflow 2.1 implementation of yolo version 3. You only look once (YOLO) is a state-of-the-art, real-time object detection system.
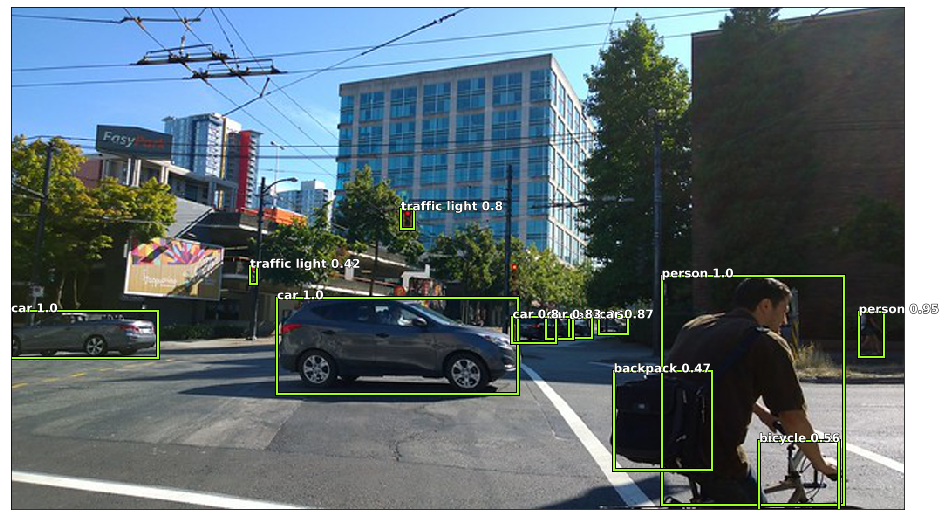
Free software: Apache Software License 2.0
Documentation: https://fabiofumarola.github.io/ultrayolo.
Features¶
Ultrayolo implements YOLO object detection paper with the following extensions:
Custom Backbones¶
Darknet: the original architecture
ResNet: ResNet50V2, ResNet101V2, ResNet152V2
DenseNet: DenseNet121, DenseNet169, DenseNet201
MobileNetV2: MobileNetV2
Focal Loss¶
Ultrayolo implements the FocalLoss for dense object detection such as RetinaNet. It can be used as alternative by changing the loss function used in the config.
model:
# The backbone for the model, default DarkNet, accepted values (DarkNetTiny, ResNet50V2, ResNet101V2, ResNet152V2, DenseNet121, DenseNet169, DenseNet201, MobileNet, MobileNetV2)
backbone: ResNet50V2
# the training mode: transfer, fine_tune, train
iou: 0.5
object_score: 0.5
reload_weights:
# accepts values focal or yolo
loss: focal
....
Customizable grid size¶
By Default YOLO used a grid system that divides the images in cells of 32, 16, and 8 pixels. Ultrayolo is modified so that it accepts values multiples of 32. That is, given an image of shape (608,608,3) it can use 32, 64, 128 and 256 as base grid. This parameter can be applied to several image shapes, but sometimes can generate mismatch in the target shape of the network and/or the dataset. The general rule is to avoid using a value of base grid size greater than image widht and height.
dataset:
# support mode singlefile, multifile, coco
mode: multifile
# the size of the image (X,X,3)
image_shape: [512, 512, 3]
# max number of objects that can be detected
max_objects: 100
# the size of the batch
batch_size: 16
# the base size of the grid in pixels used by yolo for the detection
# the standard value is 32, it can be any value but consider that it should divisible per the image shape width and height
# note that base on the values used some backbone could not be used as pretrained layers for the training
base_grid_size: 256
...
TODO¶
[X] additional backbones
[X] customizable grid-size system
[X] focal loss
[X] fully trainable using custom class numbers
[X] run configurations via yaml
[X] implemented custom metrics to monitor xy, wh, obj, no_obj and class loss
[ ] add mean average precision evaluation script
Credits¶
This package was created with Cookiecutter and the audreyr/cookiecutter-pypackage project template.